Create gym retention strategies you can be confident in

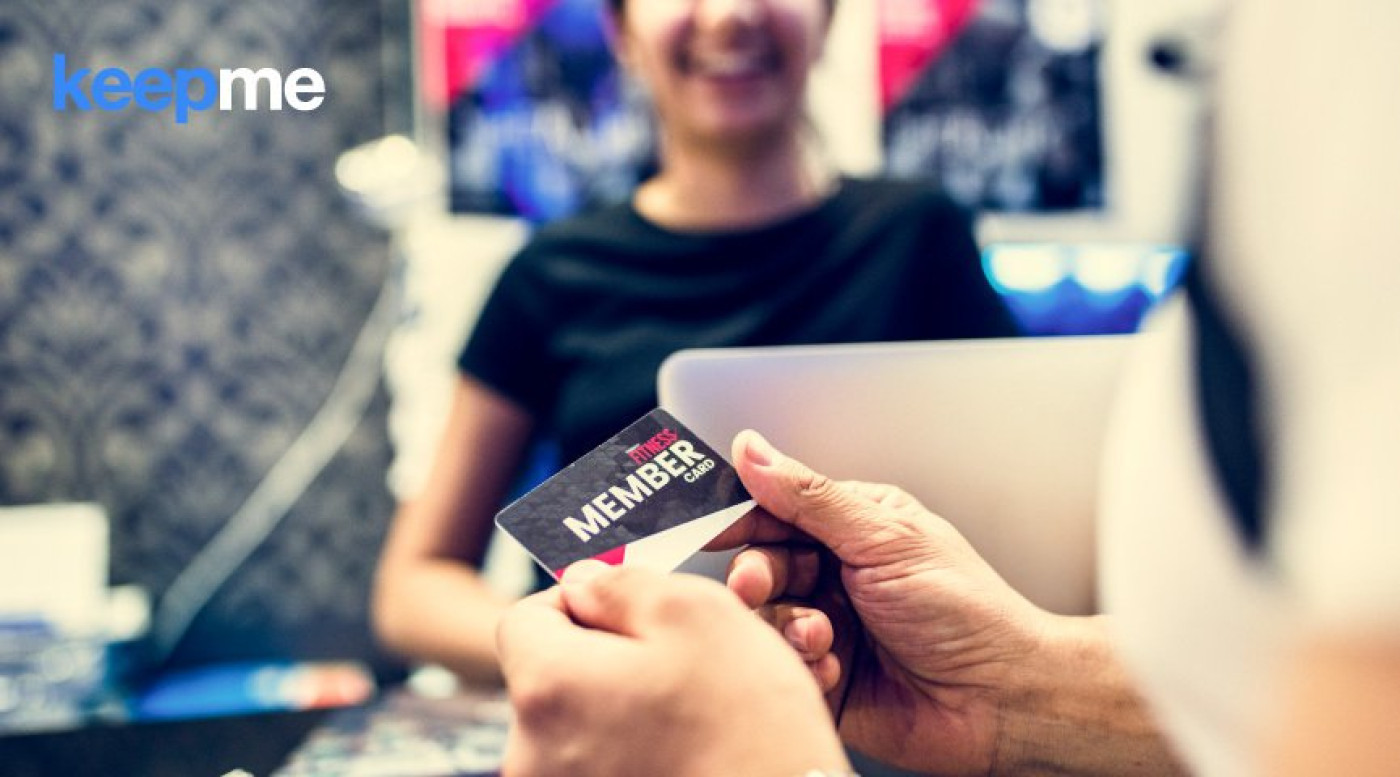
When you’re putting together forecasts for your business, there’s one thing you no doubt prize above all else. Accuracy. If you can accurately predict which of your members might leave you – just as one example, but one that’s already regularly monitored in a gym environment – you can put in place appropriate gym retention strategies.
But how accurate are your predictions right now? And what can you do to increase that accuracy – and with it, improve the impact of your gym retention strategies?
Let’s look at how you might be approaching forecasting now, and how a new approach could lead to far more transparency, accuracy, and confidence in your decision-making.
How good are your in-house experts?
How do you approach forecasting right now? Perhaps you draw on your own expertise, or that of your staff – and for rudimentary analysis, that’s OK.
Let’s highlight this with an example that will be familiar to many, whereby you look at members coming up for renewal in the next 30 days. If you then identify those who are still active, you can fairly assume there’s a strong probability they will continue.
It’s a little simplistic. It doesn’t tend to offer much in the way of a time window to try and change the predicted outcome for the better. But it serves a purpose here and forms the basis of many a gym retention strategy.
Where this approach falls down, though, is when we want to develop an understanding that spans longer periods of time. Why would we want to do this? Simple. Going back to the same member retention example, if we’re already able to identify in March those with the highest probability of leaving us in December, we then have a window in which we can try and change the outcome. This forward-looking insight would be considerably more valuable than any 30-day prediction based on even the most extensive experience.
There’s also the fact that experts, like the rest of us, are prone to cognitive distortions that materially impact the quality of the end result; prejudices, incorrect assumptions, the need to simplify, ignoring contradictions, and, of course, emotions all affect the output.
Is Business Intelligence intelligent enough?
Maybe you use a Business Intelligence (BI) platform, including that stalwart of any industry dashboard: the 12-month retention chart. Of course, it’s vital to know how your member retention breaks down by month, but what can you actually do with this information? Not a massive amount.
You can establish whether there’s an upward or downward trend. Be alerted if the previous month was considerably better or worse than the one before. But as for deliberate actions that can be driven from it and planned into your gym retention strategies, not much. The historical nature of the metric is limiting, and the one-dimensional presentation doesn’t provide many initiatives for action.
The capability to drill down would certainly make it more valuable, allowing you to explore in more depth why you got the results you got. However, what you ideally want is a forward view for the coming year – a perspective that helps you understand where you need to investigate further and that gives you time to put plans in place to mitigate.
Step forward the AI gym
Artificial intelligence (AI) addresses all the above issues, and we’ll kick things off with a big statement: while experience can certainly assist in data collection when it comes to prediction, the results secured from AI + machine learning will be many times better than anything any expert in your business could achieve.
Don’t want to accept that? Here’s just one (non-fitness) example: a LawGeex study that compared the effectiveness of lawyers versus AI in assessing the quality of non-disclosure agreements.
The study involved 20 lawyers, with decades of experience in companies such as Goldman Sachs, pitted against LawGeex’s proprietary AI platform. Participants evaluated the risks contained in five agreements by searching for 30 specific legal points.
The results of the study showed AI achieving an average accuracy of 94 percent, and maximum accuracy of 100 percent. Humans achieved 85 and 94 per cent respectively.
That might not sound wildly different but don’t underestimate the impact of small improvements in prediction accuracy. Say you have a model that lifts your accuracy from 85 to 90 per cent and another that lifts it from 98 to 99.9 per cent. The first improvement is impressive, as it means mistakes fall by a third. But in the second example, mistakes fall by a factor of 20.
Then, of course, there’s time efficiency. Returning to the LawGeex study, the lawyers required 92 minutes, on average, to complete the task. AI analyzed all the documents in 26 seconds.
Let’s take another example. Unlike chess, the game Go was considered beyond the reach of even the most sophisticated computer programs. The 4,000-year-old board game is incredibly complex, with more possible configurations for pieces than atoms in the observable universe.
In 2016, however, Go world champion Lee Se-dol was beaten 4-1 by Google’s Alpha Go. Initially trained with a dataset of 100,000 human games, Alpha Go then went on to play itself over and over, developing the ability to play the game with blinding speed. In one game, Alpha Go famously played a move that all the experts assumed was a mistake, but which went on to be the defining move of a game it subsequently won.
Grand Master Lee Se-dol later retired, saying: “Even if I become the number one, there is an entity that cannot be defeated.”
The ABC of machine learning
So, how does AI do this? How can it be so much more accurate than human experts with their years of experience?
To explain this, it’s worth a very brief detour to outline how AI works…
The majority of the predictions we use in our industry come from a branch of AI called Machine Learning. In the simplest terms, we can explain machine learning as follows: it’s when we train our AI – best thought of as a prediction machine – to predict an outcome by teaching it using data from what’s happened in the past.
By using historical data in this way, we get to understand the accuracy of our model in the set-up phase – i.e. before deployment.
Let’s say we want to predict gym sales performance over the coming months, in the hope that we can use this information to increase gym membership sales. We’d start by extracting all the historical data relating to gym sales, including all the leads, where they came from, and whether they converted or not. We’d then feed 80 per cent of this data – complete with outcomes – into our prediction machine for it to learn how an outcome is formed. Once it’s confident of its abilities, we’d then feed it the final 20 per cent of the data without the outcomes, asking it to predict these for us.
This allows us to understand how accurate the prediction machine is, and to use these initial findings to fine-tune the model if needed – for example, correcting a model when it ‘over-fits’, which is when the AI takes random fluctuations and noise found in the training data and learns these as concepts.
We then hook the AI up to the live data source so it can continue producing predictions for your business. And all the while, of course, it’s also consuming the new data and actual outcomes; even once the system is live, it keeps learning and constantly refining its output to become even more accurate (and as observed earlier, the small improvements that continue to be made can be material).
That, in a nutshell, is how machine learning works.
Critically, machine learning also looks at every data item you feed into it. It looks at every possible permutation and even develops some of its own, considering millions of permutations for every prediction it makes. Some of these will have absolutely no value. Others will represent 0.01 percent of the outcome. But when you add them all together, you get a level of accuracy that cannot possibly be replicated by a human.
Hence our bold statement earlier: that the accuracy of the predictions generated by AI and machine learning will be many times better than any expert could achieve.
The power of AI on your gym retention strategies
Let’s now look at a gym-based example – a model we ran for a UK operator back in the early days of Keepme.
This operator passed us their (somewhat patchy) data for the previous two years and held back the current year. The challenge was to identify which of the members would still be with them in six months’ time – which Keepme was able to predict with 82 per cent accuracy (before tuning, may we add, which we always do before full implementation – more on that in just a moment).
Compare this to the operator’s previous probability of accurately predicting whether a member in month six of a 12-month contract would stay or go, which was effectively a coin toss (aka no more than 51 per cent).
Even that initial jump from 51 to 82 per cent would have provided the operator with a tremendous platform to confidently target gym retention strategies towards those at risk – including members who themselves didn’t yet know they were at risk – all in a timeframe where the outcome could still be changed.
The operator now had a real-time indicator of retention probability, which would have allowed it to craft each user journey appropriately, with personalized engagement.
Delighted with the results, we sat down with the team to hear the CFO open the meeting with: “It’s not very accurate then. It’s a long way from 100 per cent.”
Let’s remind ourselves that previous accuracy was no more than 51 per cent (assuming anyone had the time to run these figures in a multi-thousand-member business). In contrast, they now had an opportunity to correctly predict retention more than eight out of every 10 times, with constant opportunities for further improvement (to reiterate, it’s all about the ‘learning’ in machine learning).
Of course, every prediction, whether human- or machine-generated, has an error possibility; the goal has to be as high a degree of accuracy as possible. But with that in mind, 82 per cent (and counting) versus 51 per cent… We may be biased, but to us, there’s a clear winner.
The nuances of prediction matter
A few final points to mention when it comes to accuracy.
First, acknowledge that what is acceptable for accuracy depends on the prediction. Forgive us if this sounds like we’re dismissing the importance of gym sales forecasts or your gym retention strategies, but presumably, everyone would agree on the impact of an incorrect diagnosis by AI analyzing an X-ray of a patient with suspected breast cancer, for example, sits in a different league.
Second, don’t call machine learning predictions into question if the results are simply not what you want to see.
And third, depending on the question being asked, understand that an incorrect prediction can actually be a good thing.
For example, a false negative for a gym operator posing a retention-focused question would see a member who was expected to leave end up staying – no bad thing, and unlikely to cause a negative impact.
A false positive, on the other hand – predicting they will not leave, when in fact they do – has more serious repercussions. That member, assumed to be ‘safe’, will generally have had no intervention, no effort made to retain them, on the basis that it wasn’t needed. In the fine-tuning of your model, these errors in gym member retention strategies would clearly need to be addressed as a priority.
At the risk of sounding simplistic, then, if you’re finding some predictions are wrong, make sure you determine if the wrong is a good wrong or a bad wrong. Some incorrect predictions have more impact than others.
Further proof of the unparalleled accuracy of AI can be found in our latest white paper – Everything You Need to Know About Data & AI – which is available for free download here.
Discover Keepme in your custom demo
Book your FREE 15-minute demo and discover how Keepme can revolutionize your sales and membership processes.